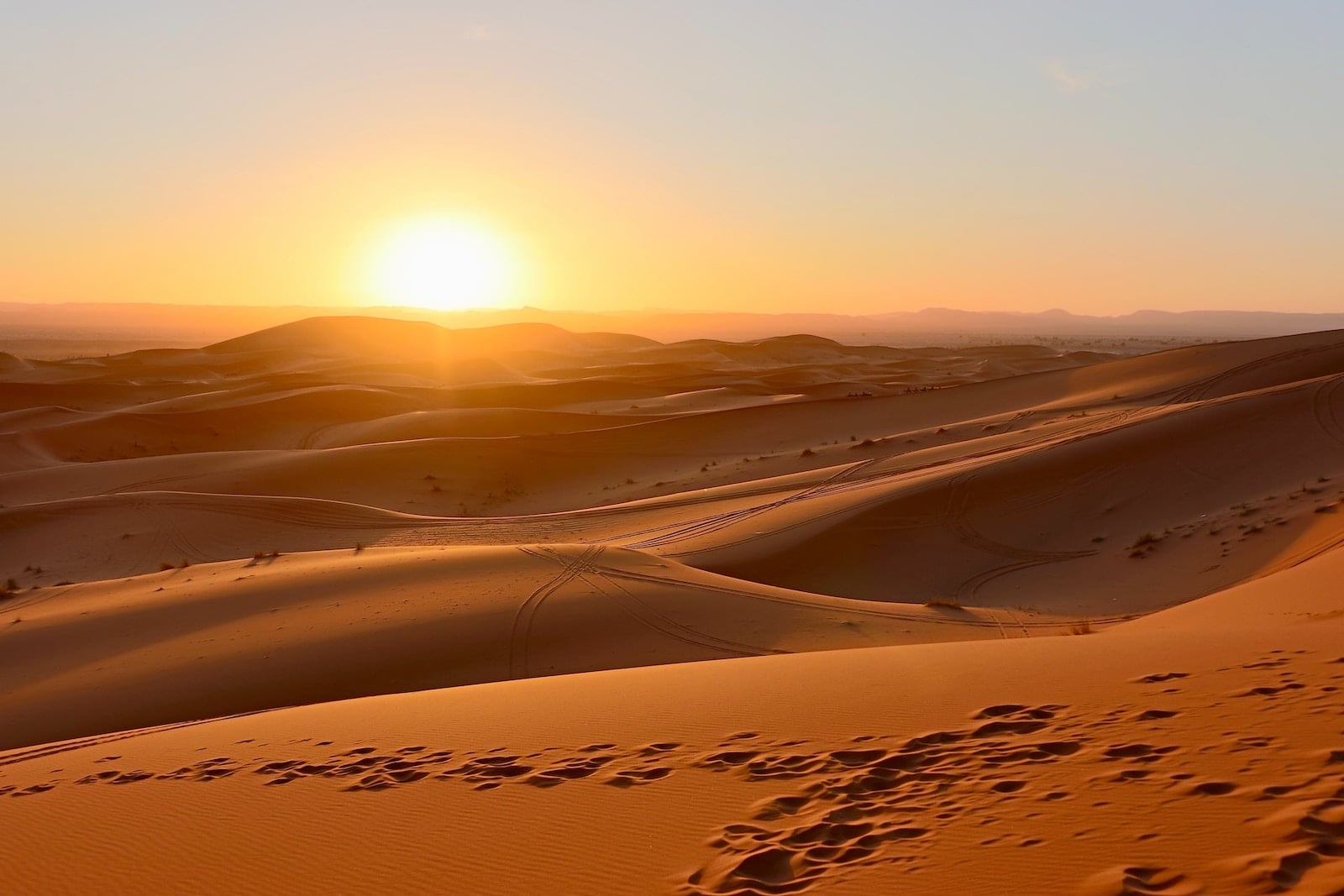
Research paper: Data deserts – compounding missing realities to no reality?
Dr Ashima Chopra | Insights, News, Women In Open Banking
16 Nov 2023
“Errors using inadequate data are much less than those using no data at all.” Charles Babbage
Data across time
When Charles Babbage invented his concept of the “difference engine” in 1923, it was primarily to “crunch” data, aimed at astronomical calculations.[1] This was followed by the “analytical engine” in 1830s, considered analogous to modern computers.[2] At that early stage of computing, Babbage had recognised that inadequate data produced fewer errors than no data.
Fast forward to the modern day and, on the one hand, very little has changed, from a computing perspective, to disagree with Babbage’s quote. On the other hand, however, everything has changed in the role data plays in contemporary times, from the days it was used for calculation of astronomical and mathematical tables.
In the world of Open Banking, Open Finance and venturing into the realm of AI, data’s value lies in what can be extracted from the data collected. Extracting “information” from the data makes the data “structured” in that it becomes organised, it becomes systematic, whereby it is “sorted” using a framework for analysis of the data. A framework that can be altered, expanded, amended, to capture and analyse the “information” the data represents, and apply this “information” to the use for which it is intended.
To ensure that data is useful, it is general practice that data (or sample of data) is representative of the population. When data collected is not representative of the population it is derived from, it leads to inadequately or inaccurately representing the population. Hence, the results of the analysis of that data will be flawed, will have errors, may represent a skewed picture of the population it is derived from or may have implicit, inherent biases, in line with how this data being analysed is collected.
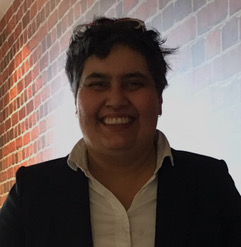
Dr Ashima Chopra
But what if data for a population cannot be collected, because data for that population does not exist? Or if data for a population is not included, as that population has not generated that data in the form the data is required? Or the population gets excluded, since it does not have sufficient data for the purpose for which it needs to be processed? Or the population gets excluded, because it does not have “visible” actions through which data can be generated for the purpose for which it is to be used?
Given that there are a large number, sometimes even infinite, of computations and permutations that can be derived from data (be it variable data or attribute data, in the form of quantitative or qualitative data), what if some populations go unrepresented, excluded because they do not have data that represents their attributes? Are there mechanisms to include these populations? Are there mechanisms to help them be part of the data revolution? Do they get “left” behind? Or does the responsibility of inclusivity only extend to what new offerings (dependent on people’s habits, for example), new use cases, new markets, increased uptake, can be produced with the least amount of accountability to populations excluded by nature of a lack of data? And, if there are enough of these new opportunities, do these exclusions matter? What is the impact, beside the economics of (production for and consumption by) these groups, of new offerings in the Open Banking, Open Finance space, when populations get left behind, when populations get excluded from the digital data canvas?
Data deserts
“Though much of the digital economics literature has focused on inequality and access and usage of the internet, algorithmic exclusion is a new and important concern for understanding digital exclusion and inequality … Algorithmic exclusion occurs when algorithms are unable to … make predictions because they lack the data to so …
Algorithmic exclusion is part of a concept known as data deserts, first written about in 2014 to describe zones which collect far less data than the average…” [3]
Algorithms are series of steps taken to solve, using data, a particular problem or generate a particular output.[4] Hence, their unequivocal importance in the realm of Open Finance or AI. An algorithm has one or more outputs, which have a specified relation to the inputs.[5] If the inputs or objects that represent reality are flawed, or missing, this “reality”, this “object”, this “data”, will be missing from the output or solution as well.
When these algorithmic exclusions, or data deserts occur, and are not proactively “bridged”, not proactively included, then what are its results, what is its impact? From a computational point of view, these are merely errors, like other biases in inputs, or data, to be rectified. But what is the “actual” impact of these? And how is this actual impact and the cost of this impact defined and calculated? And who is responsible for setting up an analytical framework to define and calculate the cost incurred?
Human progress
“The United Nations Sustainable Development Goals (SDGs) are an ambitious global initiative identifying grand challenges partitioned into 17 goals, each with specific sub-targets. The key to these goals making a real difference to people’s lives is that they are pursued in an integrated way, so that this pursuit should ‘Leave No One Behind’. [Global support for these goals was a heart-warming assertion of valuing humanity collectively with a shared commitment to acting together.] However, technological advancements in the last years have introduced a significant threat to their collective achievement, a point reiterated by the UN Secretary-General in 2020; ‘Of the SDG’s 17 goals and 169 targets, not a single one is detached from the implications and potential of digital technology…’” [6]
An enormous push for Bridging the Digital Divide (BGD) was undertaken when the Millennium Development Goals (MDGs) were put into action. The MDGs, which preceded the SDGs, failed due to the lack of critical criteria to evaluate its success of poverty reduction, as well as the inability to evaluate this in real terms. “The SDGs aim for a broader set of objectives across the full spectrum of the economic, social and environmental dimensions.”[7] And digital technologies continue to remain central for the SDGs as they did for the MDGs.
Implications of missing data
The idea of pursuing a goal in an integrated manner, leaving “no one behind”, when not adhered to, has many costly (in more than monetary terms) implications. Let’s look at one example. A non-digital example, when paper records were paramount. The results of an extensive study on Domestic Violence in 2000 showed that:
“Research to document the complex relationship between violence and … web of human rights … depends upon the existence of additional data about women seeking help. For example, relationships between violence or the threat of violence in a woman’s life and her employment status, her control of income earned, her freedom to leave the house, her freedom to meet with others, or her educational attainment are evident, but difficult to document without recorded information.”[8]
What was fascinating of this same study was the absence of data on the incident recorded, though there was a record of its occurrence. The absence of this data was, in part, due to the “belief” that the incident was not important in and by itself. It was deemed important to leave details, “data”, on the incident only if the child had been taken away from the mother.
If relevant data is not collected and represented, or data is missing, or adjustments have not been made to factor in certain “realities” that may not be represented due to non-availability of data, then it is likely that we will “create AI systems that are less likely to magnify [those qualities such as] prejudices that can skew (the AI’s) decision making.”[9]
The “marginalised”, ‘missing”, “invisible” realities get lost as the problem of missing data compounds. Those with high disposable incomes, bank accounts, travel, leisure, will find many data “homes”, such as the tourism industry, retail sector, the banking and finance sectors, all of which are data hungry. But what of the low-income groups who occupy relative or complete data deserts?
The problem compounds itself since those low in the data generating, data visible realities are not only being excluded from today’s data reality (as in when data was recorded on “paper”), but by being excluded from today’s data reality they are in danger of being excluded from the very data realities that determine tomorrow’s reality [due to training models in AI that are used for prediction and accuracy of future “events”] … into oblivion. Particularly since, “the impact of gen AI alone could automate almost 10 percent of tasks [and though] it affects all spectrums of jobs … it is much more concentrated on lower-wage jobs … [where] it will affect lower-wage workers more. It will affect people of colour more. It will affect women more. For instance, women are about 50 percent more likely to be in one of those occupations that needs to transition, compared with men [due to displacement because of AI].”[10]
Explainable AI
It is not surprising then, given the above, that there has been a demand from businesses to understand the models that drive AI, and the emergence of “Explainable AI”. Businesses want to know “how … these models derive their conclusions? What data do they use? And can … [they] … trust the results? Addressing these questions is the essence of ‘explainability,’ and getting it right is becoming essential … [which is why] many companies have begun adopting basic tools to understand how and why AI models render their insights.”[11]
It is we who build AI. Irrespective of all the regulation that may be drawn up, it is up to us, as individuals, to build the trust that needs to be establish with people regarding data and AI. It is our ethics that will dictate how the human relationship with data develops. We need to have awareness of the data we handle for AI. How is the data curated? For what purpose? To please shareholders? To attract customers? Who does it marginalise? Who does it leave behind? Whose reality does it erase? Will its predictions be fair? Will it build an honest, accurate roadmap of the future?
In the words of Fei-Fei Li, the Stanford Professor who is considered the “godmother” of AI: “AI is ‘promising’ nothing. It is people who are promising – or not promising. AI is a piece of software. It is made by people, deployed by people and governed by people.”[12]
It is, then, up to us to ensure that we design the AI that builds trust, is inclusive, not fragmented, not inaccurate. So that we build a future where we reside in a technological utopia, not a technological dystopia. Where we leave no one behind.
Dr. Ashima Chopra, B.A. (Mars Hill, USA); M.A. (Bradford, UK); M.Sc. (Bradford, UK); PGDip. (Bradford, UK); Ph.D. (Bradford, UK) is a member of Open Banking Expo’s Women in Open Banking initiative.